Master Keyword Clusters for Better SEO

Relying on individual keywords to drive your SEO strategy is increasingly insufficient. Keyword clustering has emerged as a sophisticated approach that recognises how search engines have evolved to understand topics rather than isolated terms. By properly implementing keyword clustering strategies, websites can establish stronger topical authority and create more comprehensive content experiences for users.
Keyword clustering represents the strategic organisation of semantically related search terms into coherent thematic groups. Rather than treating each keyword as an independent entity, clustering acknowledges the natural relationships between terms that users employ when searching for related information. This approach mimics how people actually think and search, moving beyond the outdated practice of keyword stuffing toward creating genuinely valuable content ecosystems.
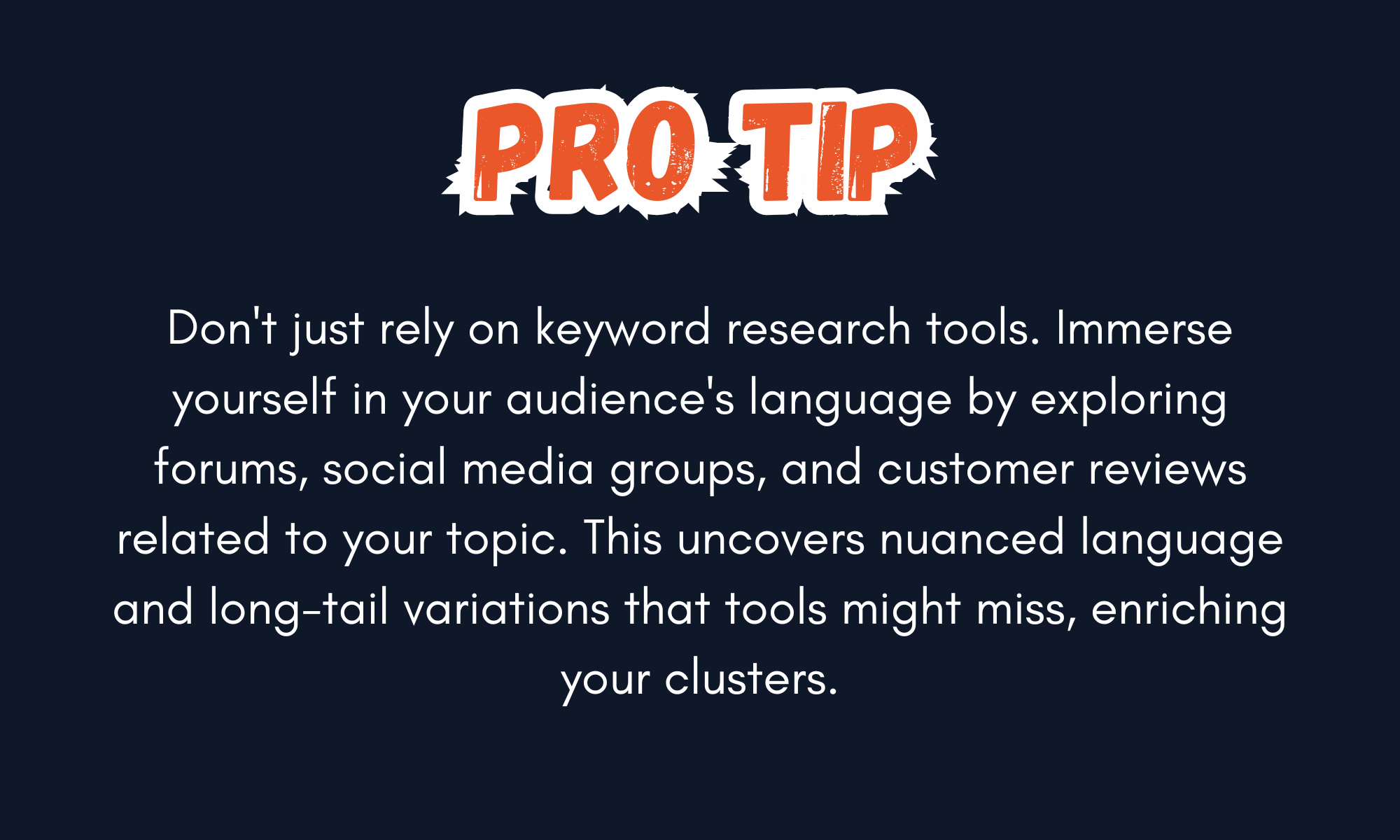
The Fundamentals of Keyword Clustering
At its core, keyword clustering involves grouping related search terms based on semantic relationships and search intent. Unlike traditional keyword research that might target single terms in isolation, clustering recognises that modern search engines understand context and related concepts.
A keyword cluster typically consists of:
- A primary target keyword (often with higher search volume)
- Semantically related secondary keywords
- Long-tail variations that address specific questions
- Conceptually adjacent terms that search engines associate together
The foundation of effective clustering lies in understanding that Google and other search engines have moved toward topic-based indexing rather than keyword-based indexing. With advancements like Google's Hummingbird, BERT, and MUM algorithms, search engines now better comprehend the relationships between terms and the contextual meaning behind searches.
For example, if your primary keyword is "sustainable gardening," your cluster might include terms like "eco-friendly gardening practices," "organic pest control methods," "water conservation in gardens," and "environmentally friendly fertilisers." These terms collectively represent a coherent topic rather than disconnected keywords.
The Strategic Value of Keyword Clustering
Implementing keyword clustering delivers multiple strategic advantages that extend beyond traditional SEO benefits:
Enhanced Topical Authority
Search engines recognise your site as an authoritative resource when your website comprehensively covers all aspects of a topic through clustered content. Rather than competing for individual keywords, you're establishing dominance over entire subject areas. This topical authority typically leads to improved rankings across all related terms within a cluster.
Improved Content Organisation
Clusters provide a logical framework for organising your content. This structure helps both users and search engines navigate your site more effectively, understanding the relationships between different pieces of content. A well-organised content structure typically leads to longer user engagement and improved crawling efficiency.
More Effective Content Planning
Working with keyword clusters reveals content gaps and opportunities that might be missed when focusing on isolated keywords. This allows content teams to develop comprehensive coverage of topics rather than producing disconnected articles that compete with each other for rankings.
Better User Experience
When visitors find content that addresses all aspects of their query, including related questions they haven't yet formulated, they receive a more satisfying user experience. This comprehensive coverage encourages longer site engagement and positions your brand as a thoughtful authority.
.png)
Methodologies for Creating Keyword Clusters
Several approaches exist for developing effective keyword clusters, ranging from manual analysis to sophisticated data-driven techniques:
Semantic Analysis
This approach involves identifying keywords with similar meanings or that belong to the same conceptual category. Modern SEO tools often provide semantic relationship data that shows how terms are connected based on their usage in search and across the web.
The process typically begins by analysing how frequently certain terms appear together in search results, identifying patterns in co-occurrence that indicate semantic relationships. Tools like SEMrush's Topic Research feature or Ahrefs' Keywords Explorer provide visualisations of these semantic connections.
Search Intent Mapping
Clustering keywords based on search intent focuses on understanding the purpose behind searches rather than just the terms themselves. This approach groups keywords that reflect similar user goals, such as:
- Informational intent ("how to grow tomatoes," "best conditions for tomato plants")
- Commercial intent ("buy tomato seedlings online," "tomato growing kits price")
- Navigational intent ("gardener's supply company tomatoes," "RHS tomato growing guide")
Grouping by intent ensures that content addresses the specific needs behind searches rather than merely matching keywords.
Data-Driven Clustering
More sophisticated approaches employ data science techniques like:
- Hierarchical clustering algorithms that group keywords based on similarity measures
- Term frequency-inverse document frequency (TF-IDF) analysis to identify related terms
- Natural language processing to understand semantic relationships
- Co-occurrence analysis of terms in top-ranking content
These data-driven methods can reveal non-obvious connections between terms and provide a more objective basis for cluster formation than intuition alone.
Technical Implementation of Keyword Clusters
Putting keyword clusters into practice requires both strategic planning and tactical implementation:
Tools for Cluster Analysis
Several tools can assist in identifying and analysing potential keyword clusters:
- Ahrefs and SEMrush provide related keywords and parent topic features
- MarketMuse offers content gap analysis based on topical relationships
- Clearscope helps identify semantically related terms for comprehensive coverage
- AnswerThePublic visualises questions related to seed keywords
- Google's Natural Language API can help identify entities and relationships
These tools help transform raw keyword data into actionable clustering insights.
Visualising Keyword Relationships
Data visualisation techniques like mind maps, force-directed graphs, and cluster diagrams can help content teams understand the complex relationships between keywords. These visual representations make abstract connections concrete and facilitate better strategic decisions about content organisation.
Implementing in Content Management Systems
For larger websites, implementing cluster-based organisation might require changes to your content management system, including:
- Creating taxonomy systems that reflect topical clusters
- Developing content templates that ensure comprehensive coverage
- Implementing tagging systems that track cluster relationships
- Establishing internal linking policies that reinforce cluster connections
These technical implementations ensure that your clustering strategy becomes embedded in your content operations rather than remaining a theoretical framework.
Content Development Based on Keyword Clusters
Translating keyword clusters into effective content requires strategic approaches to content creation:
The Pillar-Cluster Model
One of the most effective content frameworks for keyword clustering is the pillar-cluster model:
- Create comprehensive pillar content that broadly covers the main topic (e.g., "Complete Guide to Organic Gardening")
- Develop supporting cluster content that addresses specific subtopics in depth (e.g., "Organic Pest Control Methods," "Composting Techniques")
- Implement thorough internal linking between pillar and cluster content
- Update all content regularly to maintain comprehensiveness and relevance
This model creates a content ecosystem where each piece supports the others while targeting specific keyword clusters.
Content Gap Analysis
Analysing existing content against identified keyword clusters reveals opportunities for new content development. This methodical approach ensures comprehensive coverage of topics and prevents duplicative content. Regular content audits should map existing assets against keyword clusters to identify areas requiring additional development.
Internal Linking Strategy
Strategic internal linking reinforces the relationships between clustered content pieces, helping both users and search engines understand the connections. Internal links should use anchor text that reinforces the target page's primary keywords while creating logical pathways through related content.
.png)
Measuring Success and Optimisation
Evaluating the performance of keyword cluster strategies requires specific metrics and analytical approaches:
Cluster Performance Metrics
Rather than focusing solely on rankings for individual keywords, cluster performance should be evaluated holistically:
- Average position across all keywords in a cluster
- Traffic share for the topic compared to competitors
- Content coverage score (percentage of cluster keywords addressed)
- User engagement metrics for cluster-based content
- Conversion rates from cluster entry points
These comprehensive metrics provide a more accurate picture of topical authority than isolated keyword rankings.
Optimisation Strategies
Continuous improvement of keyword clusters should include:
- Refreshing content to incorporate emerging subtopics
- Expanding clusters as new related terms gain search volume
- Strengthening internal linking between related content
- Consolidating overlapping content that targets similar clusters
- Addressing content gaps identified through competitive analysis
This iterative approach ensures that keyword clusters remain relevant as search patterns evolve.
Advanced Applications and Future Trends
As search technology continues to advance, keyword clustering is evolving in several important directions:
Entity-Based SEO
Search engines increasingly understand entities (people, places, concepts, objects) rather than just keywords. Advanced clustering strategies now incorporate entity relationships, focusing on how concepts connect rather than just term similarities. This entity-based approach aligns with how modern search algorithms interpret content.
AI and Machine Learning Applications
Machine learning algorithms can identify subtle patterns in search behaviour and content performance that inform more sophisticated clustering. These technologies enable:
- Predictive keyword clustering that anticipates emerging topics
- Automated content gap analysis based on competitor coverage
- Dynamic cluster adjustments based on changing search patterns
- Personalised content recommendations within topical clusters
Voice Search Optimisation
As voice search continues to grow, keyword clusters must adapt to conversational language patterns. This includes incorporating question-based phrases and natural language variations into clusters to capture voice searches effectively.
.png)
Conclusion
Keyword clustering represents the evolution from simplistic keyword targeting to sophisticated topical authority building. By organising content around semantically related terms and comprehensive topic coverage, websites can better align with how modern search engines understand content relationships.
The most successful SEO strategies now focus on owning topics rather than ranking for keywords. This shift requires a deeper understanding of semantic relationships, user intent, and content organisation - all foundational elements of effective keyword clustering.
By implementing the clustering methodologies and content strategies outlined in this guide, digital marketers can build more coherent, authoritative content ecosystems that satisfy both search engines and users while establishing lasting topical authority.
References:
https://ahrefs.com/keywords-explorer
https://blog.google/products/search/introducing-mum/
https://blog.google/products/search/search-language-understanding-bert/
https://cloud.google.com/natural-language
https://www.marketmuse.com/about/